Kyler Sherman-Wilkins
PRB Board of Trustees, Assistant Professor of Sociology and Faculty Affiliate in the Gerontology program at Missouri State University
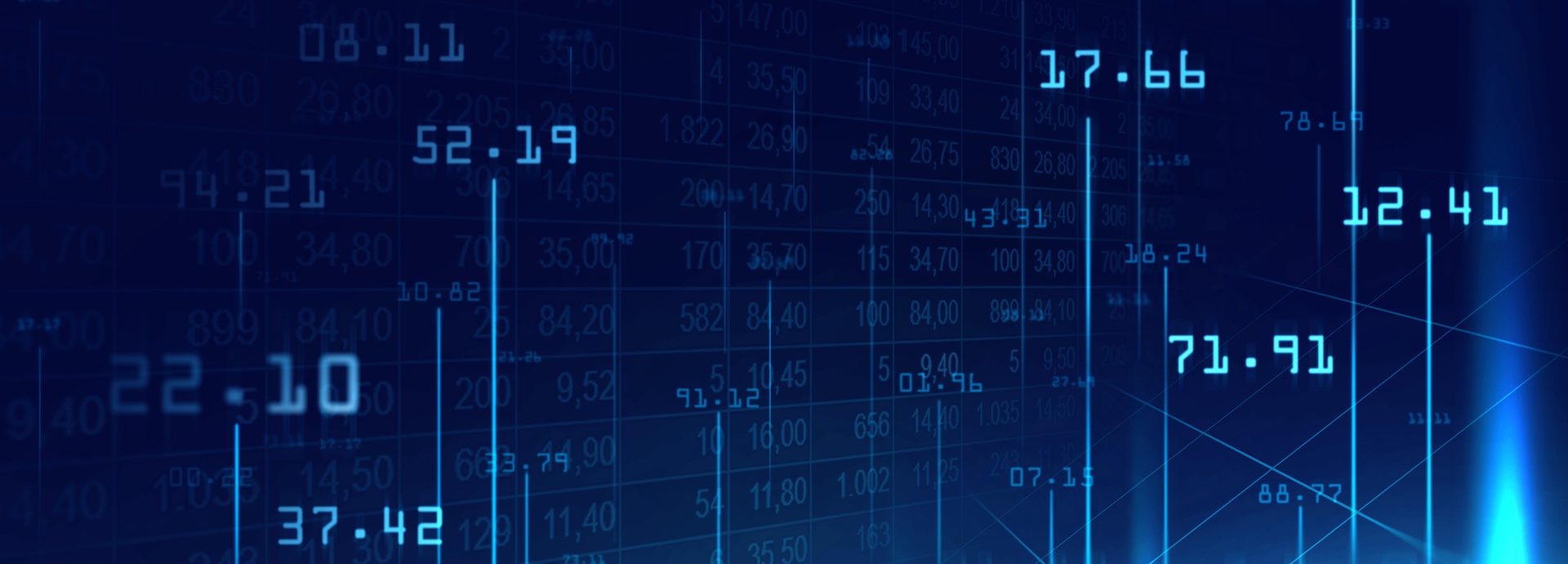
Date
June 21, 2022
Author
Teaching statistics to undergraduate social science majors with openly declared aversion to mathematics and numbers may not appear to be particularly rewarding work. While I do admit there are days when I question my life decisions as I attempt to convey the importance of the central limit theorem and confidence intervals to my pupils, I ultimately take great pride when my students begin to display the ability to use data to tell a story about the social world. One of the first lessons I teach my students is that numbers are not neutral. More accurately, the numbers generated from the analysis of data are not unaffected by the subjectivity and biases of the analyst who generates those numbers. As simple as this lesson is, it has profound implications for how we consume and interpret data. The non-neutrality of numbers generated from data can be illustrated in how data are presented, analyzed, and interpreted.
Let’s start with the presentation of data. As a concrete example, consider a jobs report released by the U.S. Bureau of Labor Statistics in June of this year. The report presents a number of data points, but by far the most important number is 3.6. It represents the number of unemployed people per 100 Americans in the labor force. Media coverage highlights this figure and notes that the unemployment rate has remained steady over the last few months. Yet, as important as this number is, it tells an incomplete story.
Another number worth considering is 6.2%, which represents the unemployment rate for Black Americans—an increase from the previous month. From April to May 2022, the unemployment rate for Black women ages 20 and older increased from 5% to 5.9%. Some other groups, including Asian Americans, experienced a decline in unemployment. Which of these numbers is most important? That’s difficult to say. While it’s important to highlight the steadiness of the U.S. unemployment rate, it is also important to note which social groups are experiencing job losses and gains.
The numbers you use depend on the story you’re trying to tell.
Numerical summaries also lack neutrality because the numbers generated from the data are the result of several choices made by the analyst producing those numbers. One of the earliest choices made by any analyst is how to appropriately measure a particular concept. Concepts like happiness, social isolation, and overall well-being are notoriously difficult to measure and quantify. Any number that indicates say, the mean level of happiness, is only useful if the measurement strategy for happiness is sound—and if the concept of happiness means the same thing across the study population.
It’s not only abstract concepts that are difficult to measure. Even supposedly straightforward categories like race, ethnicity, and social class can prove difficult to measure. In addition to settling on a measurement strategy, researchers must also make other choices. What to do with missing data? How should outliers be treated? Do certain response categories need to be collapsed? How should the analytic sample be recruited? Granted, many of these choices are decided by best practices and convention. But the inherent subjectivity of data analysis raises real doubts about the neutrality of the numbers the analyses produce.
Lastly, the neutrality of numbers is questionable because of the various ways in which they can be interpreted by the audience. As an example, I have been part of several conversations on how to address the racial/ethnic discipline gap in K-12 schools in the Springfield Public School district, the largest public school district in Missouri. The numbers indicate quite unequivocally that students of color are more likely to experience detention, suspension, and writeups, and miss more days of instructional time than their White peers. When presented with the same numbers, individuals draw very different conclusions about what explains the racial/ethnic gap. While some interpret gaps in discipline to be driven by racism and bias, others conclude the gap is due to poverty and low socioeconomic status, categories in which students of color are overrepresented.
The numbers can be viewed as telling a story that supports an individual’s preconceived notion of how the world works.
So what is a quantitative researcher to do? How do we maintain our effectiveness in communicating our numerical representations of the data given that our numbers are not neutral? Does this lack of neutrality mean that we should scrap statistical analyses? Of course not! The solution lies in acknowledging these challenges in generating numbers that represent reality and working hard to address those challenges. It means identifying the sources of bias that we as researchers have as we make choices on how to measure, analyze, and present our data. For the data consumer, it means taking a step back to ensure our interpretation of the numbers presented is not being filtered through our existing paradigm of how the world should work.
We must treat numbers that come from the analysis of data with a healthy dose of skepticism.
As I look over my teaching evaluations at the end of the semester and focus on the inevitable, albeit few, comments on how their statistics class has been the absolute worst, I cannot help but feel overjoyed at the number of students who articulate that they not only enjoyed the class more than they thought they would, but also feel that they have a better understanding of how statistics can be a useful tool to better understand the social world. Even more, they understand that while the numbers generated from statistics are powerful and can certainly shape policy, they are far from neutral and should not be treated as such.